We are excited by the endless possibilities of machine learning (ML). We recognise that experimentation is an important component of any enterprise machine learning practice. But, we also know that experimentation alone doesn’t yield business value. Organizations need to usher their ML models out of the lab (i.e., the proof-of-concept phase) and into deployment, which is otherwise known as being “in production”.
Even though organizations know that deployment is where the business value happens, model deployment is one of the first pitfalls for many organizations. This is why companies spend so much time and energy figuring out how to address this so-called “last mile” problem. The truth is that, while being able to establish an efficient way to deploy your models is important, it’s only half the equation. Once a model is deployed, ensuring peak operational performance becomes the challenge..
Organizations must think about an ML model in terms of its entire life cycle.. Continuous Operations for Production Machine Learning (COPML) helps companies think about the entire life cycle of an ML model. As we explain in our eBook, COPML is a comprehensive approach to ML model development and operation that takes a structured approach to the “ML wrangling” problems many enterprises face. Streamlining and optimising foundational activities has the knock-on effect of ensuring that ML applications deliver continuous business value for as long as possible, and that the models can be easily retired once they’ve run their useful course.
COPML accounts for the fact that true production machine learning (i.e., where the output of an ML model is integrated into the broader business environment and delivers value) sits within the wider data ecosystem and involves cross-functional stakeholders.
The Critical Role COPML Plays in Your Production ML Success
To better understand the value of COPML, let’s look at one of the most common workflows we see implemented by our clients who are actively pursuing enterprise ML projects.
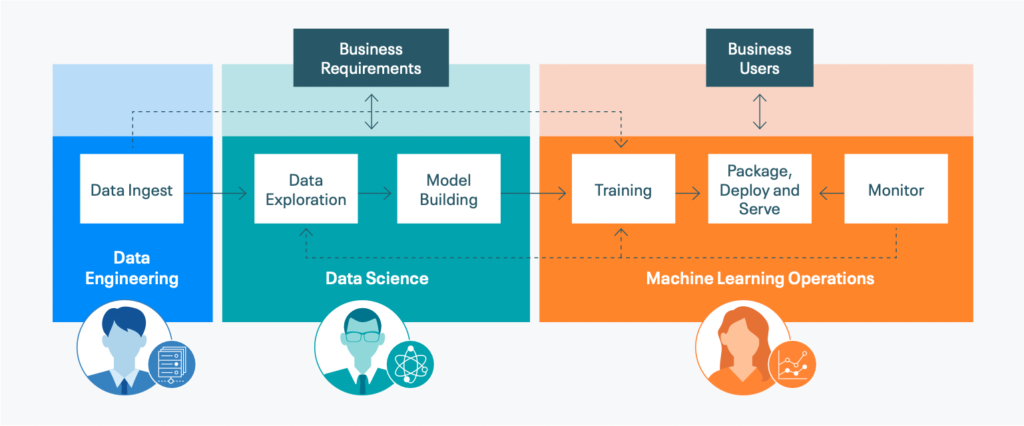
An enterprise machine learning workflow from data engineers to business users
As you can see, responsibility for enabling this workflow falls on a variety of stakeholders. This means an ML model’s development, deployment, ongoing management and, ultimately, its sustained business value, hinge on a range of cross-functional team requirements:
- Data engineers need to make sure that the data is available, clean and up to date.
- Data scientists need to perform data exploration and model building.
- ML operations team members need to manage the model to make sure it’s always available, operating accurately, and continually accessible to the relevant business applications.
If you apply this workflow to an ML use case—say, predicting customer churn or detecting pneumonia in chest x-rays—there are numerous steps involved. At any point, the cross-functional teams can disagree about what tools to use or how certain tasks should be done. Careful coordination is needed to avoid disagreements, delays, or worse, an ML model that never gets deployed.
In addition to model performance, there are also business and regulatory requirements to consider. For example, the business might have some services that require near real-time predictions and others for which time is less critical. Similarly, regulatory requirements might introduce the need for explainability and auditability. The COPML framework supports these important requirements and accounts for variations in the requirements between different ML projects. Effective machine learning projects will ensure that the processes and infrastructure deployed will support these requirements while delivering value for the business.
COPML: The Glue That Holds It All Together
Using the COPML methodology [link to eBook gated page], you can maintain production ML systems with the minimum required human input while also adhering to both specific and more intangible requirements of your production ML projects.
COPML is different to the standard approaches to software development such as the continuous integration, continuous delivery (CI/CD) framework. While CD can keep models operating, it places the emphasis on managing the developer resources and not on the automation and monitoring aspects of sustaining an ML project. CD is also first and foremost a framework for software systems. The COPML framework accounts for the needs, preferences, and actions of all stakeholders and automated processes involved in an ML workflow.
How to Implement COPML in Your Organization
Download our eBook to learn what it takes to implement COPML in your organization and the benefits of doing so. You’ll discover how to deploy ML models efficiently—and ensure that those models generate value for as long as they’re in production.